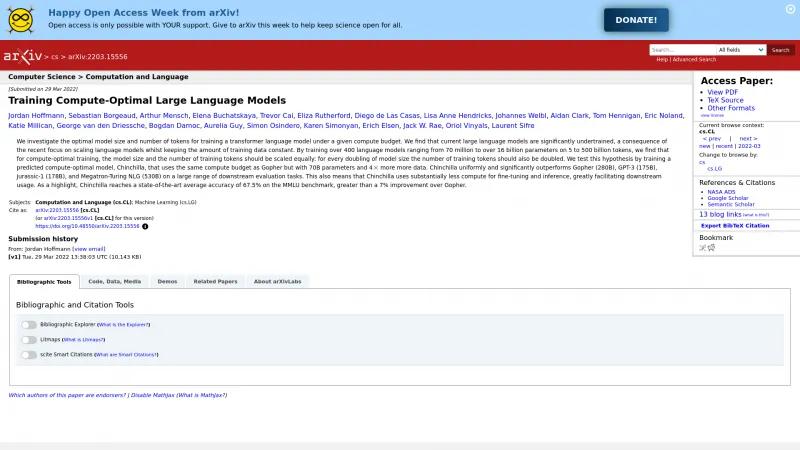
Optimize Your AI: Insights from "Training Compute-Optimal Large Language Models" by Jordan Hoffmann
Large Language ModelsDiscover how the Chinchilla model redefines training large language models by optimizing compute efficiency. Learn about its groundbreaking results and implications for AI.
About Chinchilla
The paper titled "Training Compute-Optimal Large Language Models" by Jordan Hoffmann and a team of 21 co-authors presents a groundbreaking exploration into the efficiency of training large language models. The authors meticulously analyze the relationship between model size and the number of training tokens, revealing that many current models are undertrained due to a disproportionate focus on scaling without adequate data.
One of the standout contributions of this research is the introduction of the Chinchilla model, which demonstrates that a compute-optimal approach can yield superior performance with fewer resources. By effectively doubling the training tokens alongside the model size, Chinchilla outperforms its predecessors, including Gopher and GPT-3, achieving a remarkable 67.5% accuracy on the MMLU benchmark. This not only highlights the potential for improved model training strategies but also emphasizes the importance of resource efficiency in machine learning.
The findings are not just theoretical; they have practical implications for the future of AI development, suggesting that a more balanced approach to model training could lead to significant advancements in performance while reducing computational costs. This paper is a must-read for anyone involved in the field of machine learning and natural language processing, as it challenges existing paradigms and sets a new standard for training large language models.
Leave a review
User Reviews of Chinchilla
No reviews yet.